Xudong Ma
UESTC(2018.09-2022.07)
BUAA(2022.09-)
Email: Macaronlin@buaa.edu.cn / Macaronlin@gmail.com
Google Scholar /
Github
|
|
Research
I'm interested in the direction of model quantization, and I believe that model quantization is one of the current trends in AI.
|
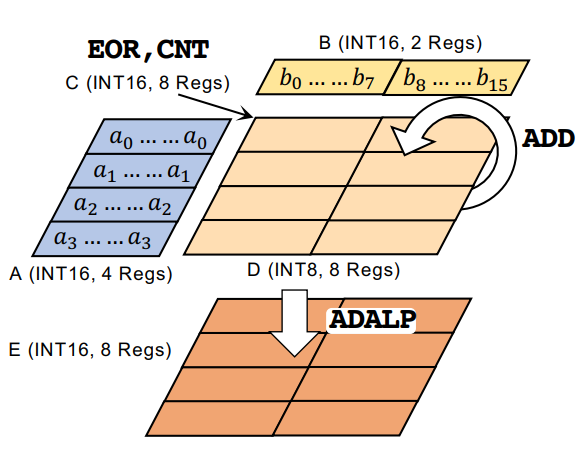 |
BiFSMN: Binary Neural Network for Keyword Spotting
[PDF]
Haotong Qin, Xudong Ma, Yifu Ding, Xiaoyang Li, Yang Zhang, Yao Tian, Zejun Ma, Jie Luo, Xianglong Liu
International Joint Conference on Artificial Intelligence (IJCAI), 2022
arXiv /
News:
(机器之心,
PaperWeekly)
In this paper, we present BiFSMN, an accurate and extreme-efficient binary network for KWS,
outperforming existing methods on various KWS datasets and achieving impressive 22.3x speedup and 15.5x storage-saving on edge hardware.
|
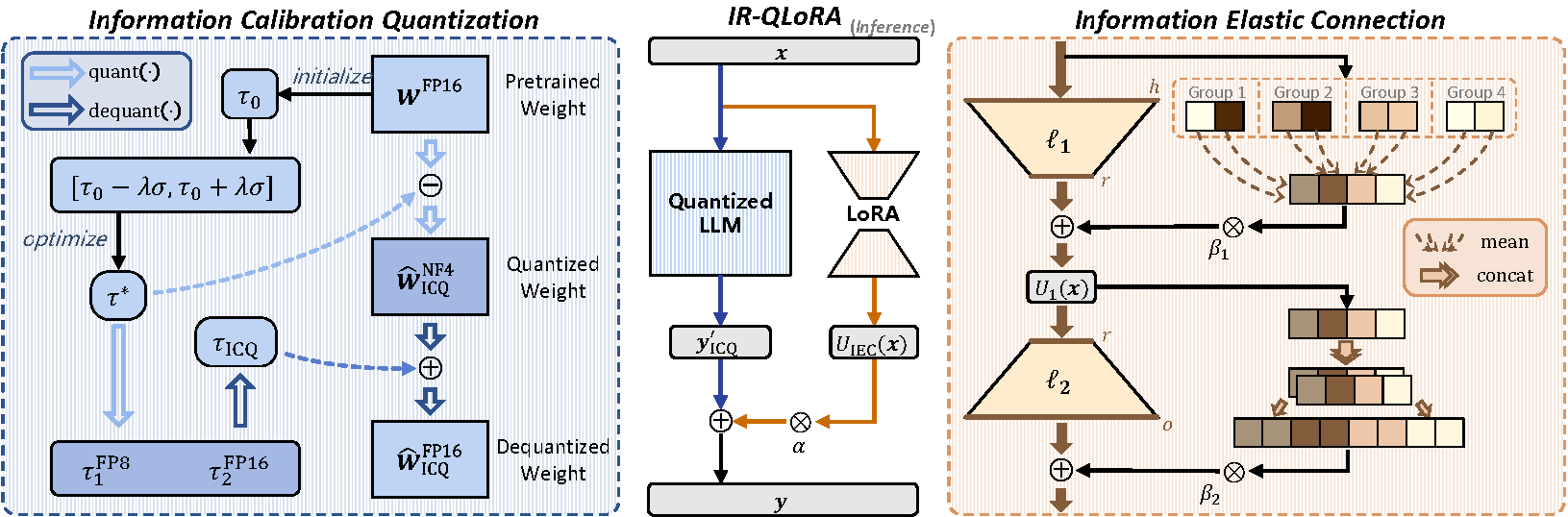 |
Accurate LoRA-Finetuning Quantization of LLMs via Information Retention
[PDF]
Haotong Qin*, Xudong Ma*, Xingyu Zheng, Xiaoyang Li, Yang Zhang, Shouda Liu, Jie Luo, Xianglong Liu, Michele Magno
International Conference on Machine Learning (ICML (oral)), 2024
arXiv /
News:
(量子位,
In this paper, we present a novel IR-QLoRA for pushing quantized LLMs with LoRA to be highly accurate through information retention. The proposed IR-QLoRA mainly relies on two technologies derived from the perspective of unified information: (1) statistics-based Information Calibration Quantization allows the quantized parameters of LLM to retain original information accurately; (2) finetuning-based Information Elastic Connection makes LoRA utilizes elastic representation transformation with diverse information.
|
|